CodeHive’s AI Readiness: Transforming Data Infrastructure for Success
At CodeHive Technologies, we are committed to transforming how organizations understand and leverage Artificial Intelligence (AI). In today’s fast-paced digital world, the ability to effectively implement AI is a critical competitive advantage. Yet, many organizations struggle with assessing their AI readiness and implementing the necessary changes to fully realize AI’s potential. Our comprehensive diagnostic assessment ensures that your organization is AI-ready.
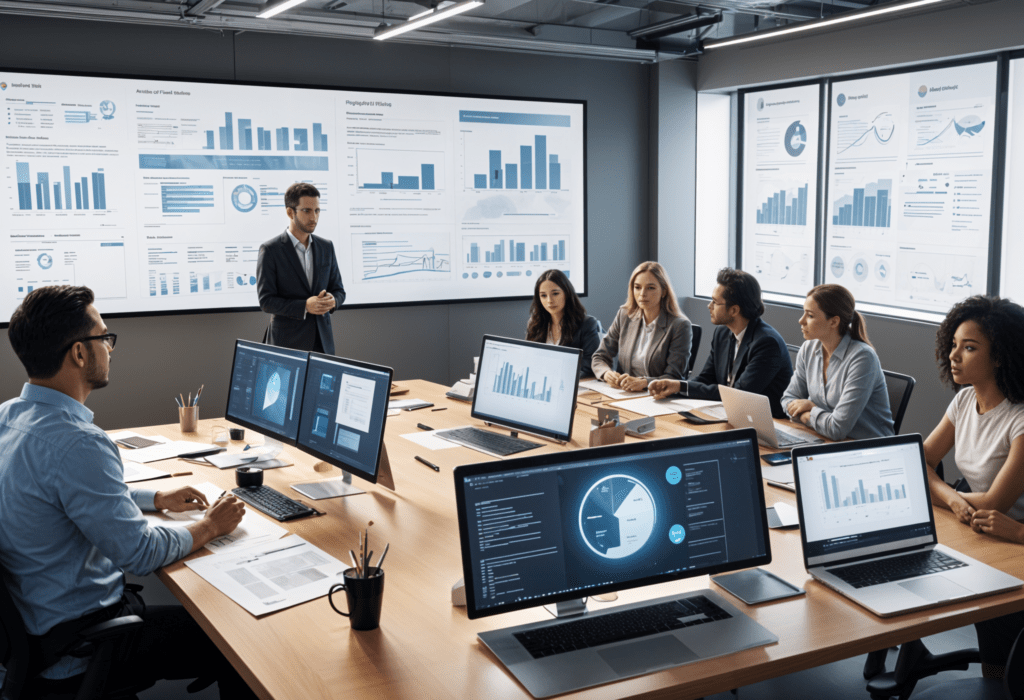
Step 1: Data and Metadata Audit
We begin with a comprehensive audit of your data and metadata. This step is crucial for identifying gaps that may hinder AI implementation. Our team meticulously examines your data assets to ensure all necessary information is captured and organized effectively. This audit serves as the foundation for understanding the current state of your data infrastructure.
Our process includes:
Inventory of Data Assets: Cataloging all data sources, databases, and repositories.
Metadata Collection: Gathering existing metadata, including data definitions, data models, and data lineage.
Data Profiling: Performing a detailed examination of data attributes, structures, and relationships.
Gap Analysis: Identifying missing, incomplete, or inconsistent metadata.
Stakeholder Interviews: Engaging with data owners and users to understand data usage, challenges, and requirements.
Documentation Review: Assessing current documentation and governance policies related to data management.
This comprehensive audit forms the foundation for understanding the current state of your data infrastructure.
Step 2: Data Quality Analysis
Next, we analyze the quality of your data. High-quality data is essential for successful AI applications. We look for inconsistencies, such as duplicate records, errors, and missing information. Our analysis highlights areas where data quality can be improved, ensuring that your data is reliable and accurate.
We analyze your data for inconsistencies and missing information through:
Data Cleansing: Detecting and correcting errors and inconsistencies within the data.
Duplicate Detection: Identifying and eliminating duplicate records.
Missing Data Handling: Addressing gaps by either imputing missing values or flagging incomplete records.
Data Validation: Verifying data accuracy through cross-referencing with trusted sources.
Consistency Checks: Ensuring data is uniform across different systems and sources.
Data Standardization: Applying consistent formats and standards to improve data interoperability.
Our detailed analysis highlights areas where data quality can be improved, ensuring reliability and accuracy.
Step 3: Metadata Enrichment
Metadata provides context to your data, making it more useful and actionable. In this step, we enhance your metadata by adding missing details and improving its overall structure. Enriched metadata enables better data management and facilitates more effective AI applications by providing the necessary context for your data.
Our enhancement process includes:
Metadata Gap Identification: Pinpointing areas where metadata is lacking or inadequate.
Attribute Enrichment: Adding additional attributes and annotations to existing metadata.
Contextual Metadata Addition: Incorporating business context and usage scenarios to enhance metadata utility.
Automated Metadata Generation: Using tools to automatically generate metadata from data sources.
Metadata Validation: Ensuring the accuracy and relevance of enriched metadata through stakeholder review.
Metadata Integration: Integrating enriched metadata into existing data management systems.
Enhanced metadata improves data management and provides the necessary context for effective AI applications.
Step 4: AI Readiness Score
Finally, we assign an AI readiness score to your organization. This score is a comprehensive measure of how prepared your organization is to implement AI solutions. It highlights both strengths and areas for improvement, offering a clear roadmap for enhancing your AI readiness. This score helps you understand your current position and guides you on the steps needed to achieve optimal AI readiness.
This process involves:
Criteria Definition: Defining key criteria and metrics for AI readiness assessment.
Scorecard Development: Creating a detailed scorecard with weighted metrics to evaluate readiness.
Data Collection: Gathering relevant data and information needed for scoring.
Assessment Execution: Applying the scorecard to evaluate the organization’s data, processes, and infrastructure.
Scoring and Analysis: Calculating the AI readiness score and analyzing results to identify strengths and weaknesses.
Reporting and Recommendations: Presenting the AI readiness score along with detailed findings and actionable recommendations for improvement.
Our AI readiness score provides a comprehensive measure of your organization’s preparedness for AI implementation, guiding you with a clear roadmap for enhancement.
Partner with CodeHive Technologies
By following this structured and detailed approach, we ensure that your organization is well-prepared to leverage AI technologies effectively. Our diagnostic assessment provides actionable insights, enabling you to make informed decisions and drive your AI initiatives forward. Partner with CodeHive Technologies to ensure your organization is AI-ready.
With CodeHive Technologies, take the first step towards transforming your data infrastructure and achieving AI excellence.
Stay tuned for the next steps!
To learn more about AI readiness assessment
Get in Touch:
Contact Us: Drop us a line here for any questions or support.
Connect on LinkedIn: Stay in the loop. Connect with us on LinkedIn for the latest insights.