Understanding Data Quality – Why It Matters for AI
In the realm of Artificial Intelligence (AI), the adage “garbage in, garbage out” holds particularly true. Data quality is paramount to the accuracy and performance of AI models. At CodeHive Technologies, we understand that high-quality data is the cornerstone of successful AI applications.
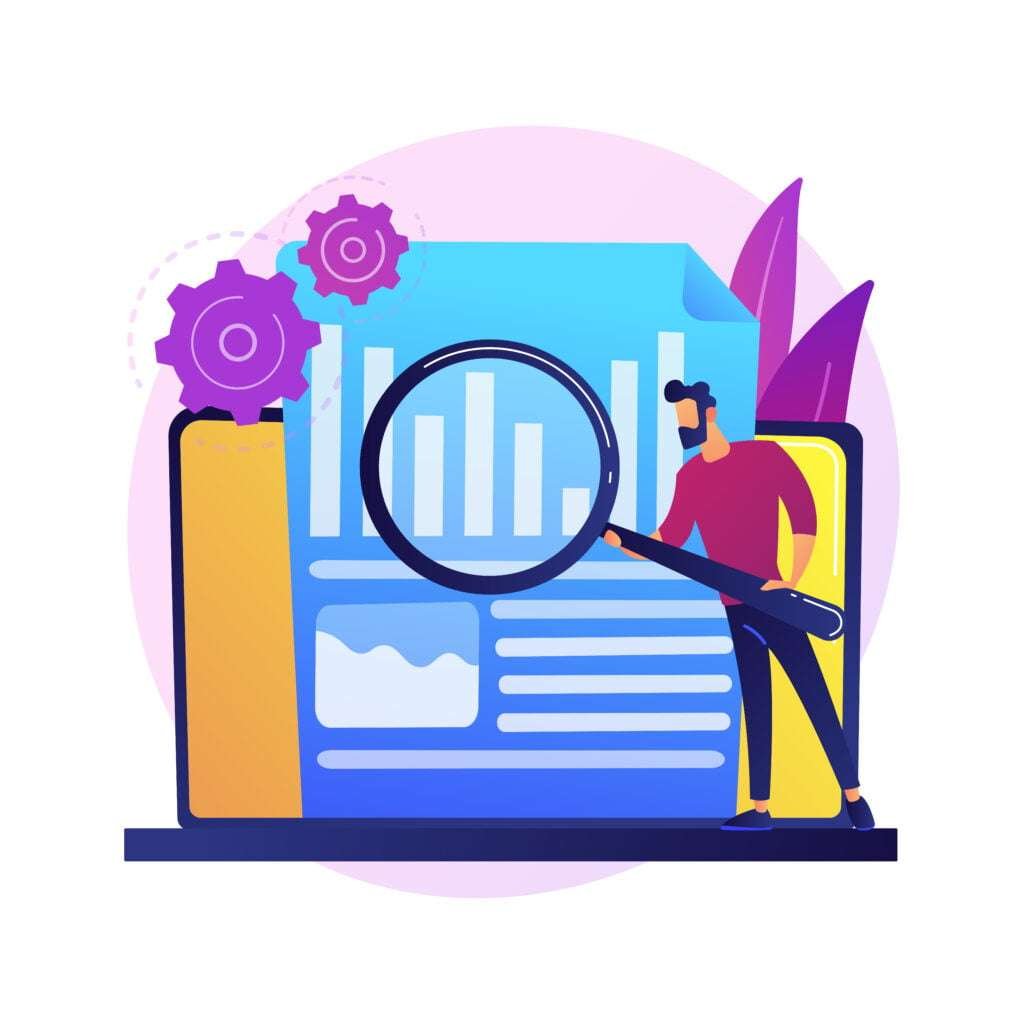
The Impact of Data Quality on AI
- Model Accuracy: AI models rely on data to learn and make predictions. Poor quality data—characterized by errors, duplicates, and missing values—can lead to inaccurate models. When models are trained on flawed data, they produce unreliable outputs, which can result in costly mistakes and misguided decisions.
- Performance: High-quality data enhances the performance of AI models. Clean, well-structured data allows models to identify patterns and relationships more effectively, leading to better performance in tasks such as classification, prediction, and clustering. In contrast, low-quality data can increase the complexity of models and slow down their processing times.
- Bias and Fairness: Data quality directly impacts the fairness of AI models. Biased or unrepresentative data can cause AI models to perpetuate existing biases, leading to unfair and discriminatory outcomes. Ensuring data quality involves checking for and mitigating biases, promoting fairness and equity in AI applications.
- Efficiency: Clean, high-quality data reduces the need for extensive preprocessing and cleaning efforts, making the data pipeline more efficient. This efficiency translates into faster development cycles and quicker deployment of AI solutions.
CodeHive Technologies’ Approach to Data Quality
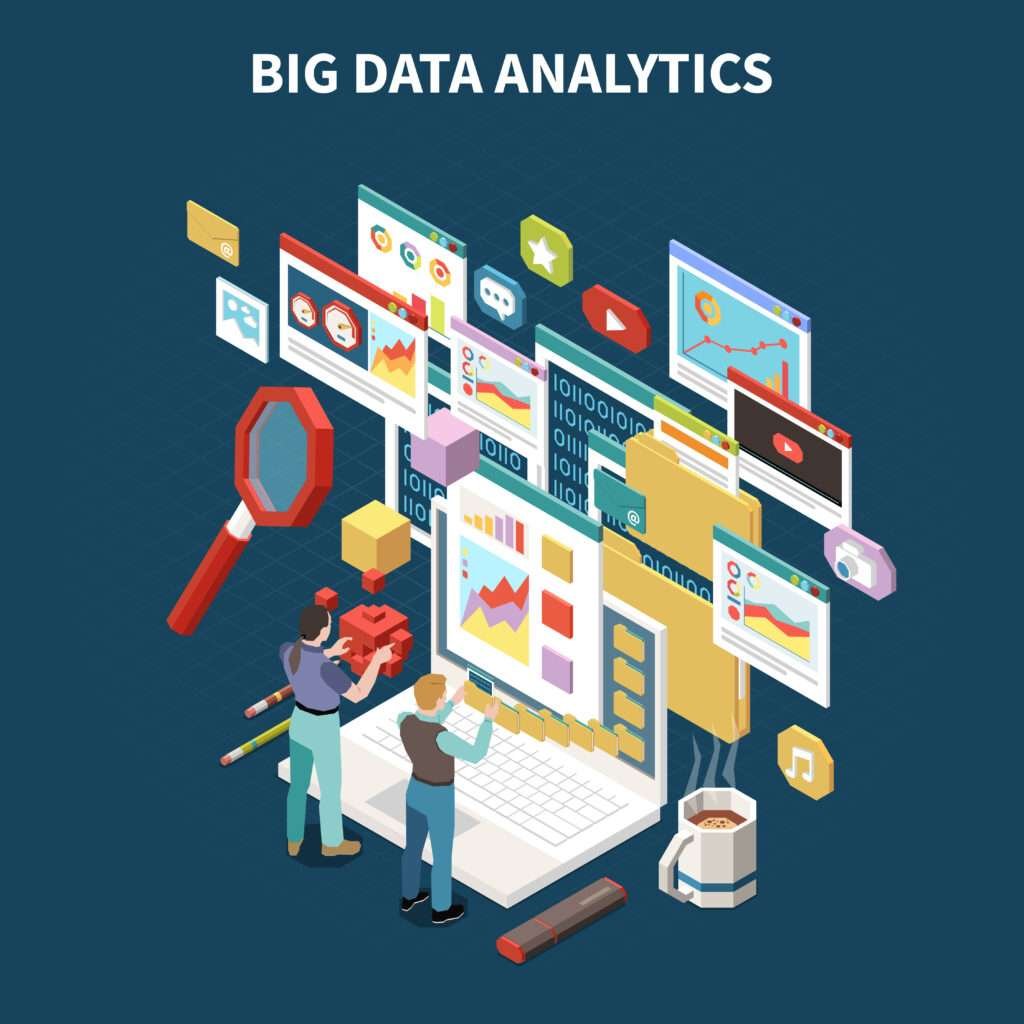
At CodeHive Technologies, we prioritize data quality through a structured approach:
Step 1: Data Cleansing We begin by detecting and correcting errors and inconsistencies within your data. This includes identifying and removing duplicate records and addressing missing values either by imputing them or flagging incomplete records.
Step 2: Data Validation Our team verifies the accuracy of your data by cross-referencing it with trusted sources. This step ensures that the data is not only clean but also reliable.
Step 3: Data Standardization We apply consistent formats and standards to your data, improving its interoperability and making it easier to integrate with other systems.
Step 4: Bias Detection and Mitigation We use advanced tools to detect biases in your data and take corrective measures to ensure fairness in AI models. This step is crucial for creating unbiased and equitable AI solutions.
High-quality data is essential for the success of AI applications. It ensures model accuracy, enhances performance, promotes fairness, and increases efficiency. At CodeHive Technologies, we are dedicated to helping organizations achieve the highest standards of data quality, ensuring that their AI initiatives are built on a solid foundation.
Stay tuned for more insights on data quality and AI!
Contact Us: Drop us a line here for any questions or support.
Connect on LinkedIn: Stay in the loop. Connect with us on LinkedIn for the latest insights.