Transform Your Data: The Artistry Of Data Cleaning For Unparalleled Insights
In the realm of data science, the journey from raw data to meaningful insights often begins with a crucial but often underestimated step—data cleaning and preprocessing. This stage is akin to preparing a canvas before a masterpiece; the cleaner and more organized the canvas, the more vivid and accurate the final picture.
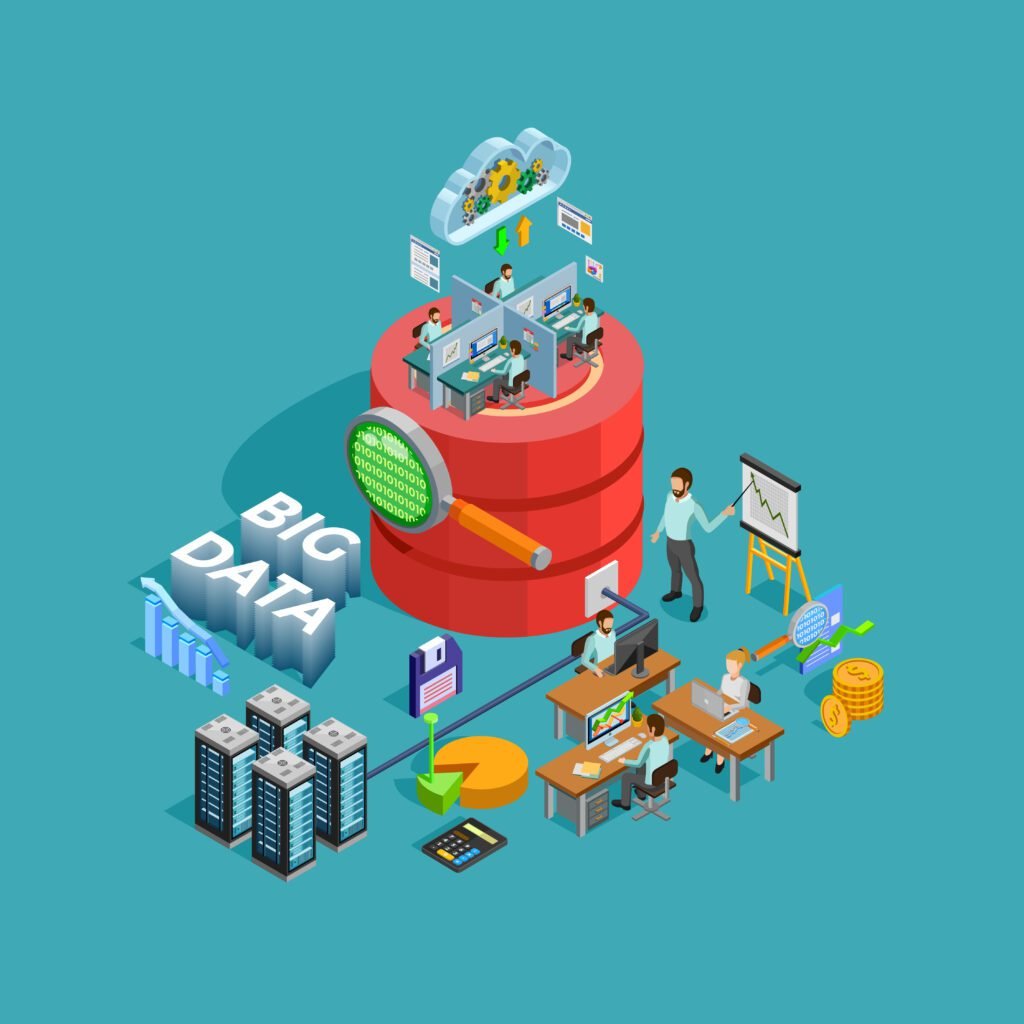
Understanding the Need:
Raw data, when collected, is rarely in the pristine form we desire. It might contain missing values, outliers, or inconsistencies that could lead our analysis astray. Data cleaning involves handling these imperfections, ensuring that our dataset is accurate, complete, and ready for analysis.
Techniques for Data Cleaning:
Handling Missing Data:
– Identification: Begin by identifying missing values in your dataset using functions like `isnull()` or `info()`.
– Imputation: Utilize methods such as mean, median, or advanced imputation techniques like K-Nearest Neighbors to fill in missing values.
– Removal: If missing values are too extensive, consider removing corresponding rows or columns strategically.
Outlier Detection and Treatment:
– Visualization: Visualize your data using box plots or scatter plots to identify potential outliers.
– Statistical Methods: Employ statistical measures like the Z-score or IQR (Interquartile Range) to detect outliers.
– Transformation: Decide whether to remove, transform, or cap outliers based on the impact on your analysis.
Data Standardization:
– Normalization: Use techniques like Min-Max scaling to bring all variables to a common scale between 0 and 1.
– Standardization: Apply Z-score normalization to ensure a mean of 0 and a standard deviation of 1.
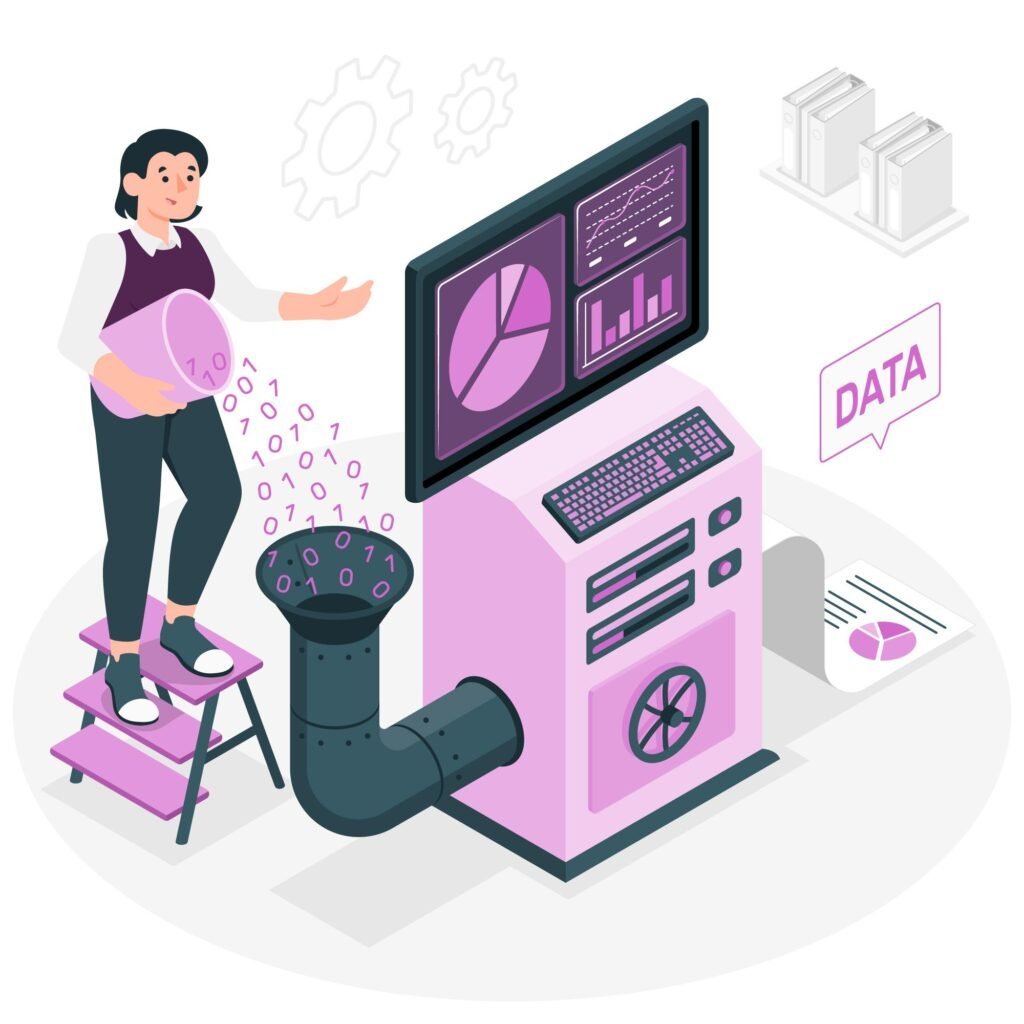
The Art of Preprocessing:
Feature Scaling:
– Normalization Techniques: Choose between Min-Max scaling, Robust scaling, or Decimal scaling based on the characteristics of your data.
– Implementation: Use libraries like Scikit-Learn in Python to easily apply scaling to your features.
Encoding Categorical Variables:
– One-Hot Encoding: Convert categorical variables into binary vectors using one-hot encoding.
– Label Encoding: Represent categorical data with integer labels, maintaining ordinal relationships.
Dealing with Imbalanced Data:
– Resampling Techniques: Explore oversampling (creating more instances of the minority class), under sampling (removing instances from the majority class), or using a combination of both.
– Synthetic Data Generation: Implement techniques like SMOTE (Synthetic Minority Over-sampling Technique) to create synthetic samples for the minority class.
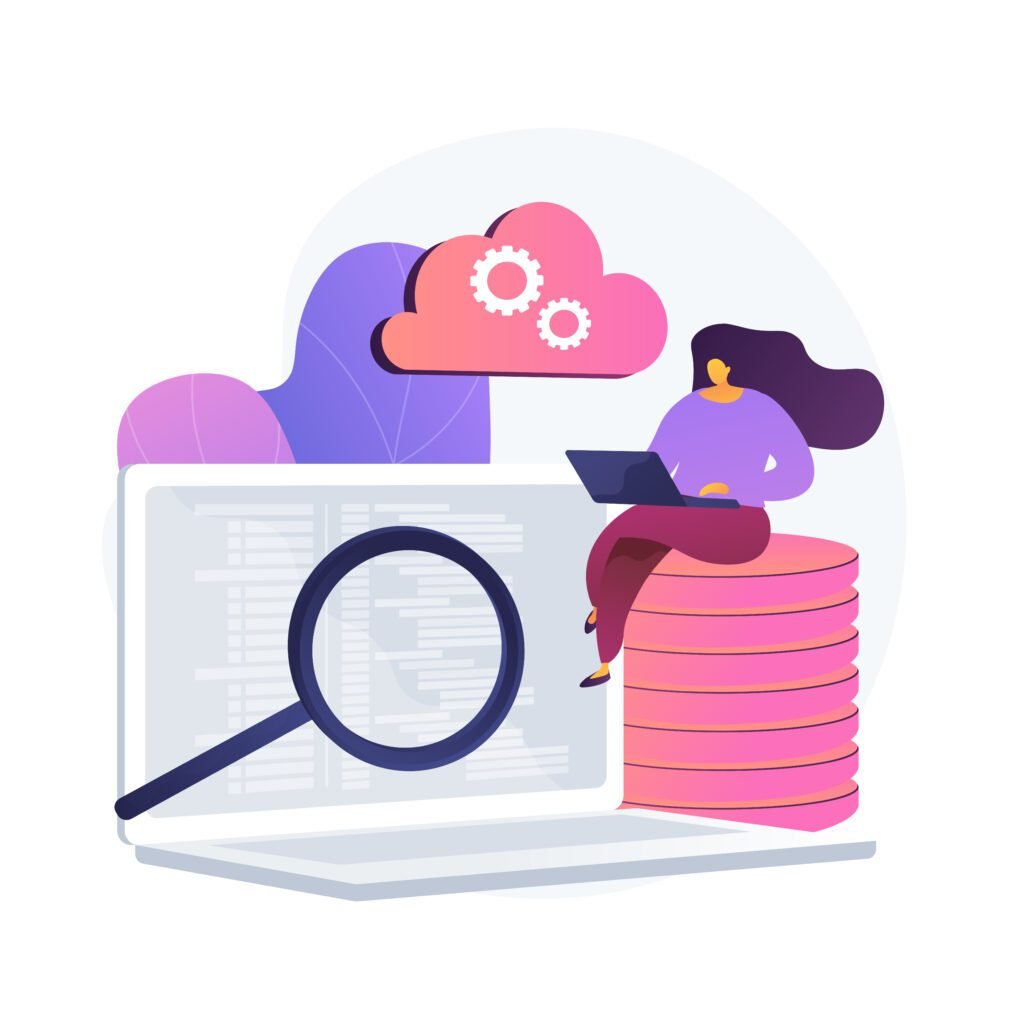
Embarking on a data science journey is akin to setting sail into a sea of possibilities, but without a well-prepared ship, the voyage can quickly become tumultuous.
Join us on this educational odyssey as we navigate the seas of data science, turning complexity into clarity and chaos into insight. By the end, you’ll not only understand the importance of this often-overlooked phase but also wield the tools to master it. Let’s embark on this transformative journey together, where data cleaning is not just a necessity but an art form in itself. Ready to elevate your data science game? Contact Us!