Adapting Data Governance to the Generative AI Challenge
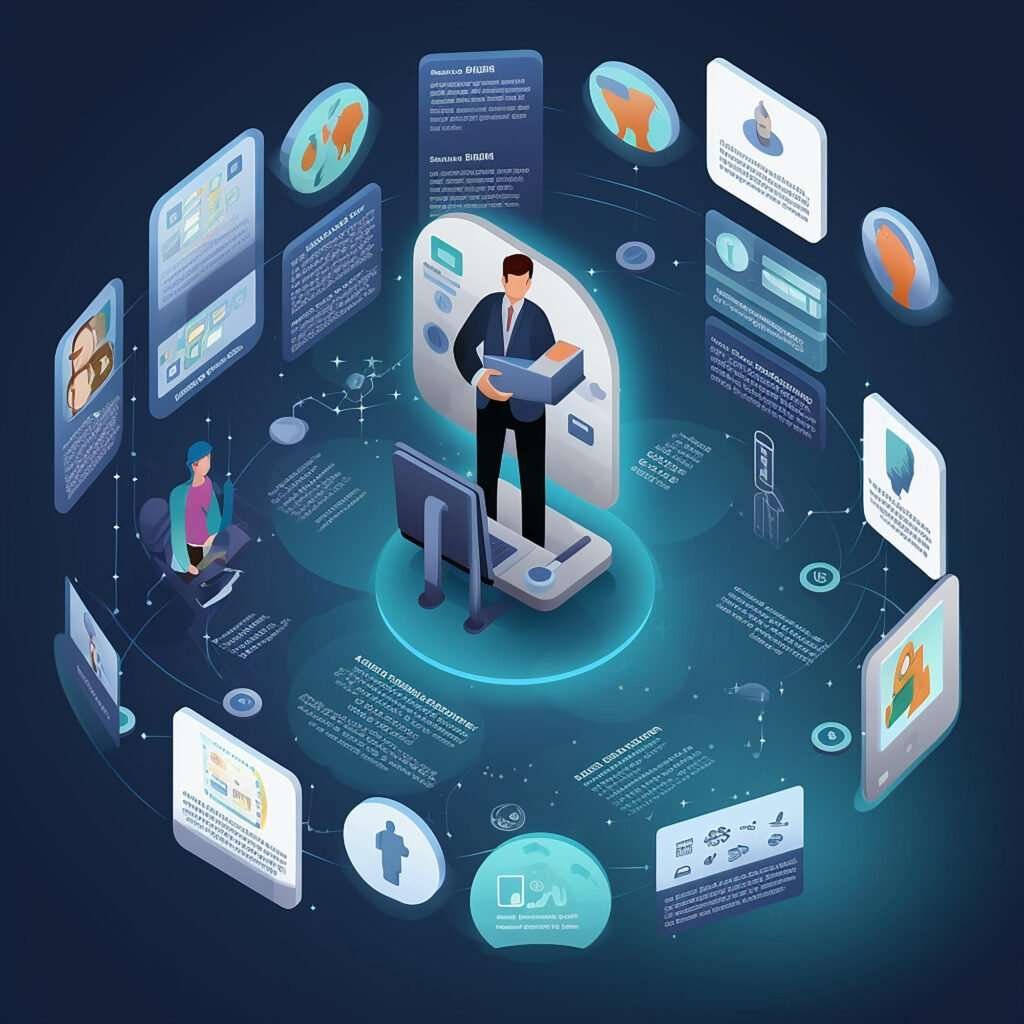
Introduction to Data Governance and Generative AI
The rise of Generative AI is revolutionizing industries. However, it presents a significant challenge for data governance. To thrive in this new era, businesses need to evolve their data governance strategies. This article explores the importance of adapting data governance to meet the challenges posed by Generative AI.
Understanding Generative AI
Generative AI models, such as GPT-3, can create content mimicking human intelligence. These models generate texts, images, and even code. They are transforming fields like marketing, entertainment, and software development. However, managing the data these AI models require introduces new governance complexities.
The Need for Evolved Data Governance
Traditional data governance frameworks focus on regulatory compliance, data quality, and security. In the era of Generative AI, these frameworks must also address new dimensions. For example, the origin, usage, and potential biases of data become critical factors. Therefore, evolving data governance is essential for harnessing AI’s full potential while mitigating its risks.
Key Challenges
- Data Origin and Provenance: Understanding where data originates from is crucial. It ensures the data’s authenticity and integrity.
- Bias and Fairness: Data used in AI models can reflect societal biases. Governing this data to ensure fairness is essential.
- Data Privacy: Generative AI requires vast amounts of data. Protecting user privacy while utilizing this data is a significant challenge.
- Model Interpretability: Ensuring that AI models are interpretable and transparent is necessary for trust.
- Compliance with Regulations: Adhering to data protection laws like GDPR is non-negotiable.
Strategies for Adapting Data Governance
To address these challenges, businesses must adopt advanced data governance strategies. Here are effective approaches:
1. Implementing Robust Data Lineage and Provenance
Tracking the full lifecycle of data is vital. Data lineage helps in understanding data’s origin, transformations, and its journey through the pipeline. Therefore, implementing robust data lineage and provenance tools is crucial.
2. Addressing Bias and Ensuring Fairness
AI can perpetuate biases present in training data. Consequently, businesses must regularly audit and assess models for biases. Adopting fairness-aware algorithms and diverse datasets can mitigate bias.
3. Ensuring Data Privacy
Protecting user privacy requires anonymizing data and following strict data protection protocols. This is especially important with regulations like GDPR and CCPA. Therefore, integrating privacy-preserving techniques is essential.
4. Enhancing Model Transparency and Interpretability
Models should be transparent and interpretable. Using explainable AI techniques helps stakeholders understand decisions made by AI. This promotes trust and accountability.
5. Complying with Regulations
Staying compliant with data protection regulations is mandatory. Regular audits and compliance checks ensure adherence to legal standards like GDPR. This prevents legal penalties and promotes ethical data use.
Leveraging Advanced Technologies
Advanced technologies can enhance data governance. For example, blockchain can provide transparent data lineage. Furthermore, differential privacy techniques can protect user data while allowing its use for AI training. Businesses should explore innovative solutions to stay ahead.
Creating a Data Governance Culture
To succeed, data governance should be a cultural cornerstone. Organizations must promote awareness and training programs. These programs should educate employees about the importance of data governance.
Promoting Accountability
Establishing clear roles and responsibilities is essential. Designating data stewards and governance officers ensures accountability. These individuals oversee governance policies and practices, ensuring compliance and effectiveness.
Fostering Collaboration
Collaboration between IT, legal, and business teams is crucial. This alignment ensures comprehensive data governance policies. Regular cross-departmental meetings can facilitate this collaboration.
In conclusion, evolving data governance to meet Generative AI challenges is imperative. With robust strategies and advanced technologies, businesses can navigate this complex landscape. Moreover, fostering a data governance culture ensures sustained compliance and ethical AI use. By embracing these changes, organizations can harness the true potential of Generative AI while ensuring data integrity and trust.
For more insights on data governance, explore best practices in data governance or learn about top data governance tools shaping the industry.
External Resource: Learn more about Generative AI on Wikipedia.